Jianyi Yang
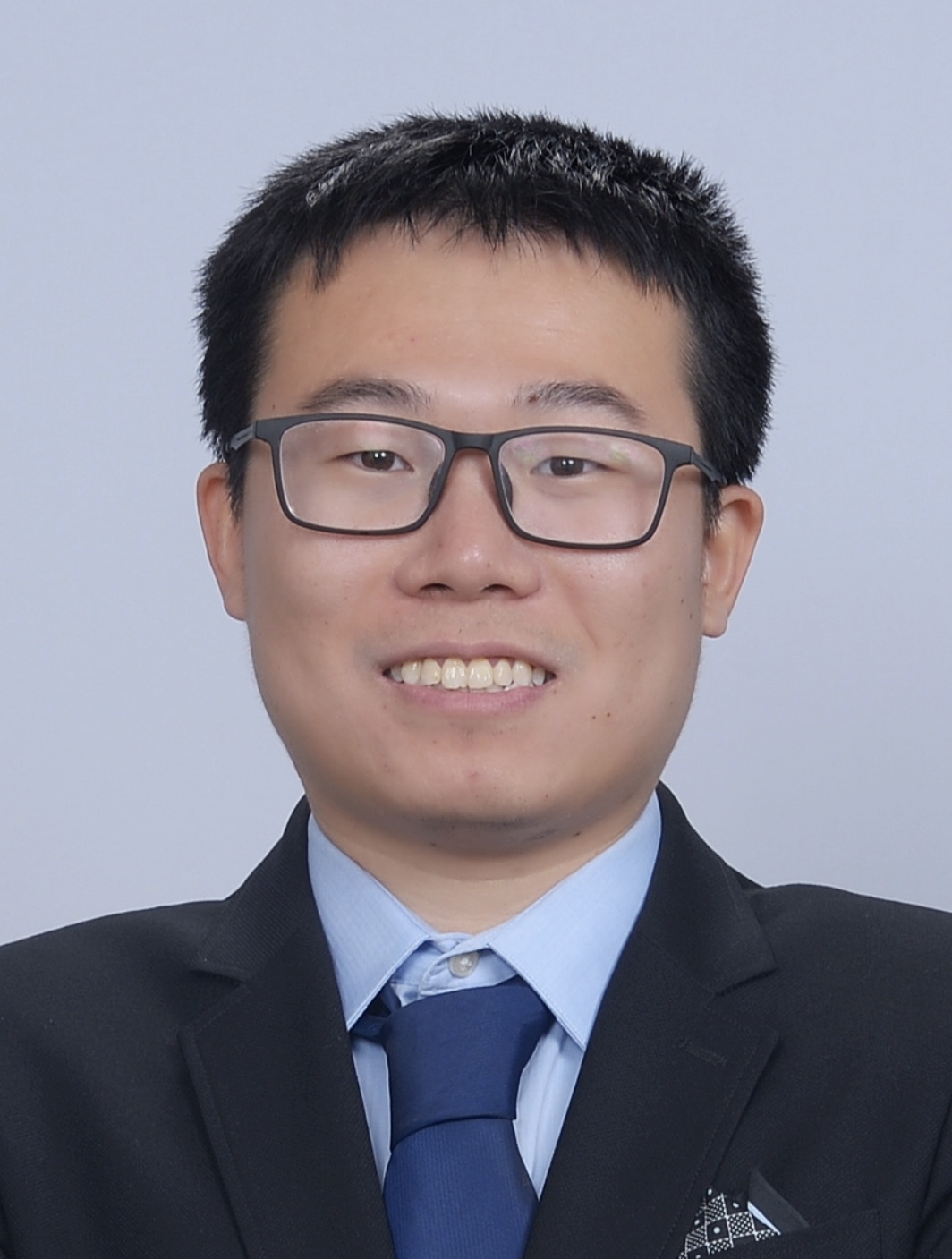
I am an Assistant Professor in the Department of Computer Science at the University of Houston. Prior to that, I was a visitor at Caltech and a postdoctoral research assistant at UC Riverside, working with Prof. Adam Wierman and Prof. Shaolei Ren. I received my PhD degree in 2023 at UC Riverside, advised by Prof. Shaolei Ren.
My research interests span AI/ML algorithms and their applications in computing systems. My research seeks to advance trustworthy and energy-efficient AI, building reliable, responsible, and sustainable AI systems. Recent research methodologies include reinforcement learning, online learning/optimization, learning-augmented algorithms, knowledge informed learning etc.
I am actively seeking self-motivated PhD students and research assistants starting Fall 2025. If you have an interest, please contact me and send me your CV and transcripts. Details in the link Openings.
news
Jan 28, 2025 | I gave a presentation on trustworthy AI in the Department of Physics at UH. Nice discussions with the colleagues from Physics! |
---|---|
Jan 19, 2025 | Our paper Learning-Augmented Online Control for Decarbonizing Water Infrastructures has been accepted by e-Energy’25! This paper provides a learning-augmented control algorithm for a critical infrastructure: the municipal water supply systems. The algorithm guarantees the worst-case safety of water supply (e.g. for fire protection) while minizing the energy costs for pumping water. |
Sep 25, 2024 | Our paper Online Budgeted Matching with General Bids has been accepted by Neurips 2024! It provides a provable algorithm to solve online budgeted matching problem with general bids. Manuscript will come out soon. |
Sep 25, 2024 | Our paper Learning-Augmented Decentralized Online Convex Optimization in Networks has been accepted by Sigmetrics’25! It proposes a novel algorithm to provably robustify machine learning predictions for decentralized optimization in networks. Our first-author Pengfei Li is on the job market this year. Pengfei is an excellent researcher and a reliable collaborator. We have worked together on a lot of works. Please reach out to him if you have position information! |
May 1, 2024 | Our paper Building Socially-Equitable Public Models was accepted by ICML 2024! Congratulations to Yejia and other co-authors! |